Elevating Restaurant Automation with Machine Learning: A Comprehensive Guide
- The Kitxens Team
- Jul 30, 2024
- 5 min read
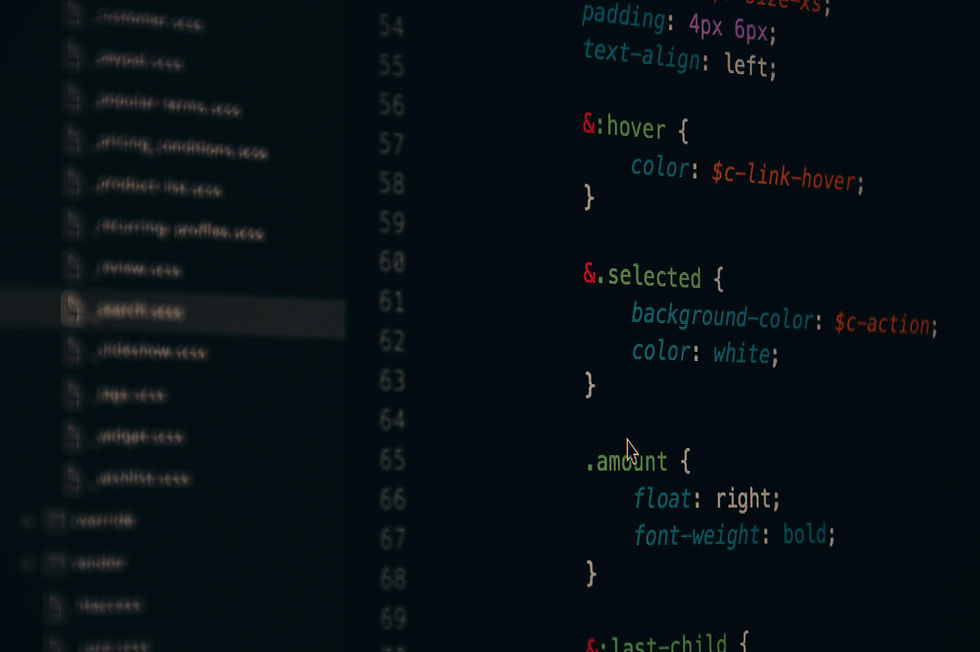
Automation in the restaurant industry is not just a fleeting trend—it's a transformative force that is reshaping how businesses operate, streamline processes, and enhance customer experiences. This article delves into how machine learning (ML) can elevate automation in restaurants, offering practical insights and real-world examples. From optimizing inventory management to enhancing customer interactions, machine learning applications in restaurants promise significant improvements in efficiency, profitability, and customer satisfaction.
Introduction to Machine Learning in Restaurants
Machine learning, a subset of artificial intelligence (AI), involves algorithms that enable computers to learn from and make decisions based on data. In the context of restaurants, ML can analyze vast amounts of data to identify patterns, predict outcomes, and automate various tasks. The integration of ML into restaurant operations can lead to more informed decision-making, reduced operational costs, and improved service quality.
Benefits of Automation in Restaurants
Enhanced Efficiency: Automation reduces manual labor, allowing staff to focus on more critical tasks.
Cost Savings: By optimizing processes, restaurants can significantly cut down on wastage and labor costs.
Improved Accuracy: Automation minimizes human errors, ensuring consistency in service and product quality.
Data-Driven Decisions: ML algorithms can analyze data to provide actionable insights for better decision-making.
Applications of Machine Learning in Restaurant Automation
1. Inventory Management
Managing inventory efficiently is crucial for any restaurant. Machine learning algorithms can predict inventory needs based on historical data, seasonal trends, and upcoming reservations. For instance, a sushi restaurant can use ML to forecast the demand for fresh fish, ensuring that they order the right amount, thus minimizing wastage and ensuring freshness.
Example: A popular pizzeria implemented an ML-based inventory system that analyzed sales data and supplier schedules to predict the exact amount of ingredients needed each day. This system reduced their ingredient waste by 25% and ensured they never ran out of key items during peak hours.
2. Dynamic Pricing
Dynamic pricing involves adjusting prices based on demand, time of day, and other factors. ML algorithms can analyze patterns and predict the best times to offer discounts or increase prices to maximize revenue.
Example: A fine dining restaurant used dynamic pricing to adjust menu prices based on reservation data and local events. This approach increased their average revenue per customer by 15% during high-demand periods without negatively impacting customer satisfaction.
3. Personalized Customer Experience
Personalization is key to enhancing customer satisfaction. ML can analyze customer preferences, past orders, and feedback to tailor recommendations and promotions. For example, a loyalty program can use ML to offer personalized discounts based on a customer's dining history.
Example: A café chain used ML to personalize their loyalty program, sending targeted promotions based on individual customer preferences and purchasing habits. This personalized approach increased their customer retention rate by 20%.
4. Predictive Maintenance
Maintaining kitchen equipment is critical for smooth operations. ML can predict when equipment is likely to fail based on usage patterns and maintenance records, allowing restaurants to perform preventive maintenance and avoid costly breakdowns.
Example: A large fast-food chain implemented predictive maintenance for their fryers and grills. By predicting when parts would need replacement, they reduced equipment downtime by 30% and saved thousands in repair costs.
5. Workforce Management
Scheduling staff effectively is a complex task that can benefit from automation. ML can analyze past sales data, seasonal trends, and employee availability to create optimal schedules that ensure adequate staffing during peak times while avoiding overstaffing during slow periods.
Example: A busy urban restaurant used an ML-based scheduling system that adapted to changing demand patterns. This system reduced labor costs by 10% and improved employee satisfaction by providing more predictable work schedules.
6. Customer Feedback Analysis
Analyzing customer feedback manually can be time-consuming. ML algorithms can process and categorize feedback from various sources, such as social media, review sites, and customer surveys, to identify common issues and areas for improvement.
Example: A national restaurant chain used ML to analyze customer reviews and social media comments. The insights gained from this analysis helped them address recurring issues quickly, leading to a 15% increase in positive reviews over six months.
7. Menu Optimization
Determining which menu items to keep, modify, or remove can be challenging. ML can analyze sales data, customer preferences, and market trends to provide recommendations for menu optimization.
Example: A casual dining restaurant used ML to analyze their menu performance, identifying underperforming items and suggesting new dishes based on current food trends. This data-driven approach increased their overall sales by 12%.
8. Fraud Detection
In the financial aspects of restaurant operations, ML can help detect fraudulent activities, such as fake discount codes or unusual payment patterns, protecting the business from potential losses.
Example: A restaurant group implemented an ML-based fraud detection system that flagged suspicious transactions in real-time. This system prevented several instances of fraud, saving the company substantial amounts of money.
9. Supply Chain Optimization
Optimizing the supply chain ensures that restaurants receive their ingredients on time and at the best prices. ML can analyze supplier performance, delivery schedules, and market prices to optimize orders and supplier selection.
Example: A high-end restaurant used ML to streamline their supply chain, ensuring timely delivery of fresh produce while negotiating better prices with suppliers. This optimization reduced their food costs by 8%.
10. Marketing Campaigns
Effective marketing is essential for attracting and retaining customers. ML can analyze customer data to create targeted marketing campaigns, ensuring that promotions reach the right audience at the right time.
Example: A restaurant used ML to segment their customer base and create personalized email marketing campaigns. These targeted campaigns had a 25% higher open rate and a 20% higher conversion rate compared to generic promotions.
11. Demand Forecasting
Predicting customer demand accurately helps in planning resources and inventory. ML algorithms can analyze various factors such as weather, local events, and historical data to forecast demand.
Example: A seaside restaurant used demand forecasting to adjust their staffing and inventory levels based on predicted weather conditions and local events. This proactive approach reduced wastage and ensured they could handle peak crowds efficiently.
12. Voice Assistants
Voice assistants powered by AI can enhance customer service by taking orders, answering queries, and providing recommendations. Integrating voice technology can streamline operations and improve customer satisfaction.
Example: A drive-thru fast-food restaurant implemented a voice assistant to take orders. This technology reduced wait times, improved order accuracy, and increased overall customer satisfaction.
13. Chatbots
Chatbots can handle customer inquiries, take reservations, and provide information about the menu and promotions. They can operate 24/7, ensuring that customers always have access to the information they need.
Example: A high-traffic restaurant integrated a chatbot on their website and social media platforms. The chatbot handled 60% of customer inquiries, freeing up staff to focus on in-person guests and improving overall service efficiency.
14. Virtual Reality Training
Training staff using virtual reality (VR) can provide immersive and hands-on experience without the risks associated with real-world training. VR can simulate various scenarios, helping staff to be better prepared for actual service.
Example: A large restaurant chain used VR to train new employees on safety protocols and customer service techniques. This innovative training method reduced onboarding time by 30% and improved trainee confidence and performance.
15. Table Management Systems
Automated table management systems can optimize seating arrangements, reduce wait times, and improve customer flow. These systems can also integrate with online reservations to provide a seamless dining experience.
Example: A busy brunch spot implemented an automated table management system that dynamically adjusted seating based on real-time data. This system reduced wait times by 20% and increased table turnover rates, boosting revenue.
The integration of machine learning and automation in the restaurant industry is transforming the way businesses operate, making them more efficient, cost-effective, and customer-focused. From inventory management to personalized marketing, the applications of ML are vast and varied, offering numerous opportunities for restaurants to enhance their operations and deliver exceptional customer experiences. By embracing these technologies, restaurants can stay ahead in a competitive market, ensuring long-term success and profitability.
Comments